2019 Journal Issues January 1, 2019
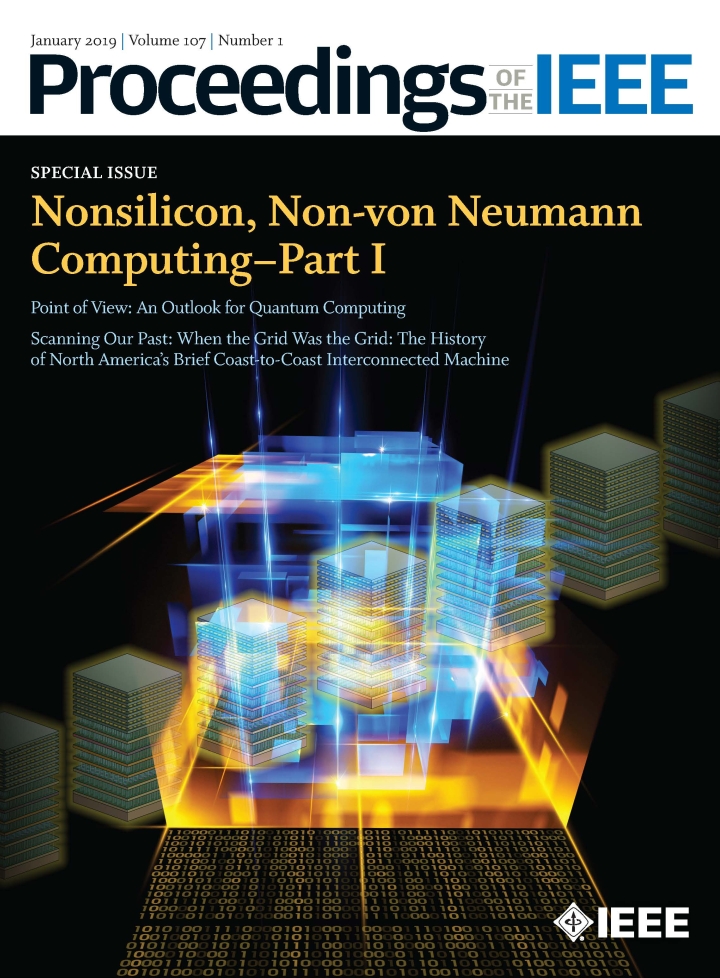
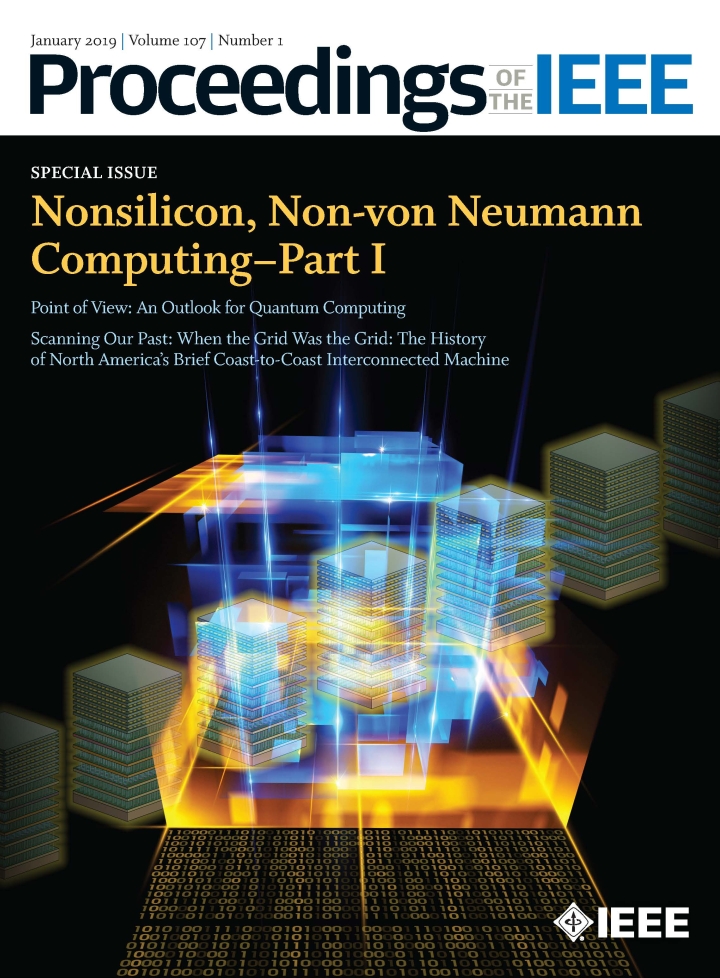
Special Issue: Nonsilicon, Non-von Neumann Computing–Part I
Volume 107, Issue 1
January 2019
Guest Editors
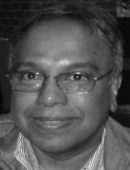
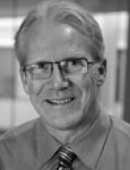
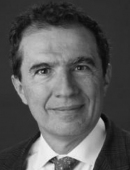
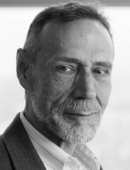
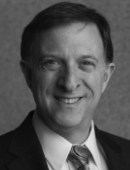
Special Issue Papers
By S. Basu, R. E. Bryant, G. De Micheli, T. Theis, and L. Whitman
Novel Materials and Devices
By M. M. Sabry Aly, T. F. Wu, A. Bartolo, Y. H. Malviya, W. Hwang, G. Hills, I.Markov, M. Wootters, M.M. Shulaker, H.-S. P. Wong, and S.Mitra
This paper enables energy-efficient computing for transformative abundant-data applications through heterogeneous integration of energy-efficient logic devices immersed in dense nonvolatile memory, with fine-grained connectivity in a monolithic 3-D architecture.
By J. C. Wong and S. Salahuddin
This paper provides an overview of a groundbreaking theoretical and experimental work on this promising new type of field-effect transistor.
By D. Carmean, L. Ceze, G. Seelig, K. Stewart, K. Strauss, and M. Willsey
This paper attempts to address the problem of long-term storage and retrieval of large volumes of data based on emerging DNA technology.
Physics-Based Non-von Neumann Paradigm
By A. Raychowdhury A. Parihar, G. H. Smith, V. Narayanan, G. Csaba, M. Jerry, W. Porod, and S. Datta
This paper discusses a computing architecture inspired by physics, via the radically different approach of using arrays of oscillators.
By N. R. Shanbhag, N. Verma, Y. Kim, A. D. Patil, and L. R. Varshney
This paper considers a principled information-theoretic approach to the design of non-von Neumann architectures via statistical computing which leverages information-based metrics.
Neuromorphic Paradigm
By W. Haensch, T. Gokmen, and R. Puri
This paper explores the current state of neuromorphic deep learning architectures in silicon CMOS technology.
By A. Rahimi, P. Kanerva, L. Benini, and J. M. Rabaey
This paper takes an unconventional approach to learning machines based on little explored but much promising notion of hyperdimensional computing.
By A. Neckar, S. Fok, B. V. Benjamin, T. C. Stewart, N. N. Oza, A. R. Voelker, C. Eliasmith, R. Manohar, and K. Boahen
This paper provides an overview of a current approach for the construction of a programmable computationing machine inspired by the human brain.
CMOS and High-Performance Computing
By E. Testa, M. Soeken, L. G. Amarù, and G. De Micheli
This paper provides a state-of-the-art view on the status of logic design flows in conventional silicon CMOS as well as using several of the emerging technologies.
By J. Cong, Z. Fang, M. Huang, P. Wei, D. Wu, and C. H. Yu
This paper deals with the important issue of specialization in designing computing hardware that can potentially provide at least a near-term strategy to combat Moore’s law slowdown.
By G. P. Fettweis, M. Dörpinghaus, J. Castrillon, A. Kumar, C. Baier, K. Bock, F. Ellinger, A. Fery, F. H. P. Fitzek, H. Härtig, K. Jamshidi, T. Kissinger, W. Lehner, M.Mertig, W. E. Nagel, G. T. Nguyen, D. Plettemeier, M. Schröter, and T. Strufe
This paper describes a leading European effort on applications of basic technologies to energy-efficient servers and high-performance computing of the future, that has been ongoing for more than a decade.
Point of View
By H. J. Trussell
Point of View
By D. Maslov, Y. Nam, and J. Kim
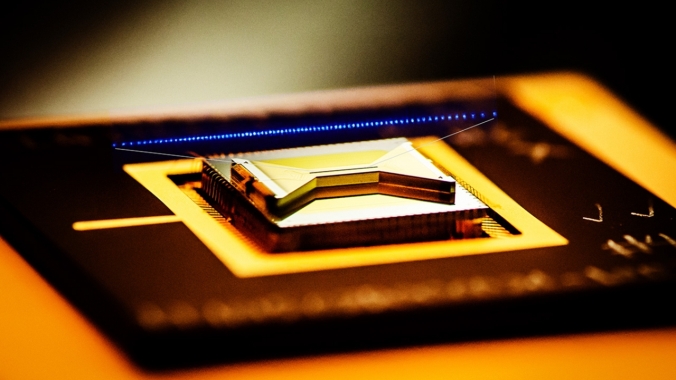
1 Comment
Comments are closed.